Multilingual natural language processing (NLP) is a rapidly advancing field that aims to develop language models capable of understanding & generating text in multiple languages. These models facilitate effective communication and information access across diverse linguistic backgrounds. This field’s importance lies in its potential to bridge the gap between different language speakers, making technological advancements in AI accessible globally. However, developing such models presents significant challenges due to the complexities of handling multiple languages simultaneously.
One of the main issues in multilingual NLP is the predominant focus on a few major languages, such as English and Chinese. This narrow concentration results in a significant performance gap for models when applied to less commonly spoken languages. Consequently, many languages still need to be represented, limiting AI technologies’ applicability and fairness. Addressing this disparity requires innovative approaches to enhance the quality and diversity of multilingual datasets, ensuring that AI models can perform effectively across a broad spectrum of languages.
Traditional methods for improving multilingual language models often involve translating preference data from English to other languages. While this strategy helps somewhat, it introduces several problems, including translation artifacts that can degrade model performance. Relying heavily on translation can lead to a lack of diversity in the data, which is crucial for robust model training. Collecting high-quality multilingual preference data through human annotation is a potential solution, but it is both expensive and time-consuming, making it impractical for large-scale applications.
Researchers from Cohere For AI have developed a novel, scalable method for generating high-quality multilingual feedback data. This method aims to balance data coverage and improve the performance of multilingual large language models (LLMs). The research team introduced a unique approach that leverages diverse, multilingual prompts and completions generated by multiple LLMs. This strategy not only increases the diversity of the data but also helps avoid the common pitfalls associated with translation artifacts. The models used in this research include Cohere’s Command and Command R+, specifically designed for multilingual capabilities.
The methodology involves translating approximately 50,000 English prompts into 22 additional languages using the NLLB 3.3B model. These prompts are then used to generate completions in each language, ensuring high diversity and quality in the data. The research team also compared completions generated directly in the target language to those translated from English, finding that the former significantly reduced the occurrence of translation artifacts. This approach resulted in a diverse set of multilingual preference pairs crucial for effective preference optimization.
The performance of the preference-trained model was evaluated against several state-of-the-art multilingual LLMs. The results were impressive, with the preference-trained model achieving a 54.4% win rate against Aya 23 8B, the current leading multilingual LLM in its parameter class. Additionally, the model showed a 69.5% win rate or higher against other widely used models such as Gemma-1.1-7B-it, Meta-Llama3-8B-Instruct, and Mistral-7B-Instruct-v0.3. These results highlight the effectiveness of the researchers’ approach in improving the performance of multilingual LLMs through enhanced preference optimization.
Further analysis revealed that increasing the number of languages in the training data consistently improved the model’s performance. For example, training with five languages resulted in a win rate of 54.9% on unseen languages, compared to 46.3% when training only in English. Moreover, online preference optimization methods, such as Reinforcement Learning from Human Feedback (RLHF), proved more effective than offline methods like Direct Preference Optimization (DPO). The online techniques achieved higher win rates, with RLOO outperforming DPO by a margin of 10.6% in some cases.

In conclusion, the research conducted by Cohere For AI demonstrates the critical importance of high-quality, diverse, multilingual data in training effective multilingual language models. The innovative methods introduced by the research team address the challenges of data scarcity and quality, resulting in performance improvements across a wide range of languages. The study not only sets a new benchmark for multilingual preference optimization but also underscores the value of online training methods in achieving superior cross-lingual transfer and overall model performance.
Check out the Paper. All credit for this research goes to the researchers of this project. Also, don’t forget to follow us on Twitter.
Join our Telegram Channel and LinkedIn Group.
If you like our work, you will love our newsletter..
Don’t Forget to join our 46k+ ML SubReddit
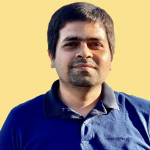
Asif Razzaq is the CEO of Marktechpost Media Inc.. As a visionary entrepreneur and engineer, Asif is committed to harnessing the potential of Artificial Intelligence for social good. His most recent endeavor is the launch of an Artificial Intelligence Media Platform, Marktechpost, which stands out for its in-depth coverage of machine learning and deep learning news that is both technically sound and easily understandable by a wide audience. The platform boasts of over 2 million monthly views, illustrating its popularity among audiences.