Multimodal models aim to create systems that can seamlessly integrate and utilize multiple modalities to provide a comprehensive understanding of the given data. Such systems aim to replicate human-like perception and cognition by processing complex multimodal interactions. By leveraging these capabilities, multimodal models are paving the way for more sophisticated AI systems that can perform diverse tasks, such as visual question answering, speech generation, and interactive storytelling.
Despite the advancements in multimodal models, current approaches still need to be revised. Many existing models cannot process and generate data across different modalities or focus only on one or two input types, such as text and images. This leads to a narrow application scope and reduced performance when handling complex, real-world scenarios that require integration across multiple modalities. Further, most models cannot create interleaved content—combining text with visual or audio elements—thus hindering their versatility and utility in practical applications. Addressing these challenges is essential to unlock the true potential of multimodal models and enable the development of robust AI systems capable of understanding and interacting with the world more holistically.
Current methods in multimodal research typically rely on separate encoders and alignment modules to process different data types. For example, models like EVA-CLIP and CLAP use encoders to extract features from images and align them with text representations through external modules like Q-Former. Other approaches include models like SEED-LLaMA and AnyGPT, which focus on combining text and images but do not support comprehensive multimodal interactions. While GPT-4o has made strides in supporting any-to-any data inputs and outputs, it is closed-source and lacks capabilities for generating interleaved sequences involving more than two modalities. Such limitations have prompted researchers to explore new architectures and training methodologies that can unify understanding and generation across diverse formats.
The research team from Beihang University, AIWaves, The Hong Kong Polytechnic University, the University of Alberta, and various renowned institutes, in a collaborative effort, have introduced a novel model called MIO (Multimodal Input and Output), designed to overcome existing models’ limitations. MIO is an open-source, any-to-any multimodal foundation model capable of processing text, speech, images, and videos in a unified framework. The model supports the generation of interleaved sequences involving multiple modalities, making it a versatile tool for complex multimodal interactions. Through a comprehensive four-stage training process, MIO aligns discrete tokens across four modalities and learns to generate coherent multimodal outputs. The companies developing this model include M-A-P and AIWaves, which have contributed significantly to the advancement of multimodal AI research.
MIO’s unique training process consists of four stages to optimize its multimodal understanding and generation capabilities. The first stage, alignment pre-training, ensures that the model’s non-textual data representations are aligned with its language space. This is followed by interleaved pre-training, incorporating diverse data types, including video-text and image-text interleaved data, to enhance the model’s contextual understanding. The third stage, speech-enhanced pre-training, focuses on improving speech-related capabilities while maintaining balanced performance across other modalities. Finally, the fourth stage involves supervised fine-tuning using a variety of multimodal tasks, including visual storytelling and chain-of-visual-thought reasoning. This rigorous training approach allows MIO to deeply understand multimodal data and generate interleaved content that seamlessly combines text, speech, and visual information.
Experimental results show that MIO achieves state-of-the-art performance in several benchmarks, outperforming existing dual-modal and any-to-any multimodal models. In visual question-answering tasks, MIO attained an accuracy of 65.5% on VQAv2 and 39.9% on OK-VQA, surpassing other models like Emu-14B and SEED-LLaMA. In speech-related evaluations, MIO demonstrated superior capabilities, achieving a word error rate (WER) of 4.2% in automatic speech recognition (ASR) and 10.3% in text-to-speech (TTS) tasks. The model also excelled in video understanding tasks, with a top-1 accuracy of 42.6% on MSVDQA and 35.5% on MSRVTT-QA. These results highlight MIO’s robustness and efficiency in handling complex multimodal interactions, even when compared to larger models like IDEFICS-80B. Also, MIO’s performance in interleaved video-text generation and chain-of-visual-thought reasoning showcases its unique abilities to generate coherent and contextually relevant multimodal outputs.
Overall, MIO presents a significant advancement in developing multimodal foundation models, providing a robust and efficient solution for integrating and generating content across text, speech, images, and videos. Its comprehensive training process and superior performance across various benchmarks demonstrate its potential to set new standards in multimodal AI research. The collaboration between Beihang University, AIWaves, The Hong Kong Polytechnic University, and many other renowned institutes has resulted in a powerful tool that bridges the gap between multimodal understanding and generation, paving the way for future innovations in artificial intelligence.
Check out the Paper. All credit for this research goes to the researchers of this project. Also, don’t forget to follow us on Twitter and join our Telegram Channel and LinkedIn Group. If you like our work, you will love our newsletter..
Don’t Forget to join our 50k+ ML SubReddit
Want to get in front of 1 Million+ AI Readers? Work with us here
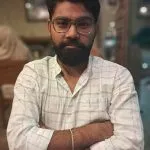
Nikhil is an intern consultant at Marktechpost. He is pursuing an integrated dual degree in Materials at the Indian Institute of Technology, Kharagpur. Nikhil is an AI/ML enthusiast who is always researching applications in fields like biomaterials and biomedical science. With a strong background in Material Science, he is exploring new advancements and creating opportunities to contribute.