Atmospheric science and meteorology have recently made strides in modeling local weather and climate phenomena by capturing fine-scale dynamics crucial to precise forecasting and planning. Small-scale atmospheric physics, including the intricate details of storm patterns, temperature gradients, and localized events, requires high-resolution data to be accurately represented. These finer details play an important role in applications ranging from daily weather forecasts to regional planning for disaster resilience. Emerging technologies in machine learning have paved the way for creating high-resolution simulations from lower-resolution data, enhancing the capacity to predict such details and improving regional atmospheric modeling.
One major challenge in this area is the significant difference between the resolution of large-scale data inputs and the higher resolution needed to capture fine atmospheric details. Data for large-scale weather patterns often comes in coarse formats that fail to encapsulate the finer nuances required for localized predictions. The variability between large-scale deterministic dynamics, such as broader temperature changes, and smaller, more stochastic atmospheric features, such as thunderstorms or localized precipitation, complicates the modeling process. Furthermore, the limited availability of observational data exacerbates these challenges, restricting the capacity of existing models and often leading to overfitting when attempting to represent complex atmospheric behaviors.
Traditional approaches to addressing these challenges have included conditional diffusion and flow models, which have achieved significant results in generating fine details in image processing tasks. These methods, however, need to improve in atmospheric modeling, where spatial alignment and multi-scale dynamics are particularly complex. In previous attempts, residual learning techniques were used to model the deterministic components first, followed by super-resolving residual details to capture small-scale dynamics. This two-stage approach, though valuable, introduces risks of overfitting, especially with limited data, and needs mechanisms to optimize both deterministic and stochastic elements of atmospheric data. Consequently, many existing models need help to balance these components effectively, especially when dealing with large-scale, misaligned data.
To overcome these limitations, a research team from NVIDIA and Imperial College London introduced a novel approach called Stochastic Flow Matching (SFM). SFM is designed specifically to address the unique demands of atmospheric data, such as the spatial misalignment and complex multi-scale physics inherent in weather data. The method redefines data input by encoding it to a latent base distribution closer to the target fine-scale data, allowing for improved alignment before applying flow matching. Flow matching creates realistic small-scale features by transporting samples from this encoded distribution to the target distribution. This approach allows SFM to maintain high fidelity while mitigating overfitting, achieving superior robustness compared to existing diffusion models.
SFM’s methodology comprises an encoder that translates coarse-resolution data into a latent distribution that mirrors the fine-scale target data. This process captures deterministic patterns, a foundation for adding small-scale stochastic details through flow matching. To handle uncertainties and reduce overfitting, SFM incorporates adaptive noise scaling—a mechanism that dynamically adjusts noise in response to the encoder’s error predictions. By leveraging maximum likelihood estimates, SFM balances deterministic and stochastic influences, refining the model’s capacity to generate fine-scale details with greater accuracy. This innovation provides a well-adjusted method to accommodate variability within the data, allowing the model to respond dynamically and prevent over-reliance on deterministic information, which could otherwise lead to errors.
The research team conducted comprehensive experiments on synthetic and real-world datasets, including a weather dataset from Taiwan’s Central Weather Administration (CWA). The results demonstrated SFM’s significant improvement over conventional methods. For example, in the Taiwan dataset, which involves super-resolving coarse weather variables from 25 km to 2 km scales, SFM achieved superior results across multiple metrics such as Root Mean Square Error (RMSE), Continuous Ranked Probability Score (CRPS), and Spread Skill Ratio (SSR). For radar reflectivity, which requires entirely new data generation, SFM outperformed baselines by a notable margin, demonstrating improved spectral fidelity and precise high-frequency detail capture. Regarding RMSE, SFM maintained lower errors than baselines, while the SSR metric highlighted that SFM was better calibrated, achieving values close to 1.0, indicating an optimal balance between spread and accuracy.
The SFM model’s superiority was further illustrated through spectral analysis, where it closely matched the ground truth data across various weather variables. While other models, such as conditional diffusion and flow matching techniques, struggled to achieve high fidelity, SFM consistently produced accurate representations of small-scale dynamics. For instance, SFM effectively reconstructed high-frequency radar reflectivity data—absent from input variables—illustrating its capacity to generate new, physically consistent data channels. Moreover, SFM achieved these results without compromising calibration, demonstrating a well-calibrated ensemble that supports probabilistic forecasting in uncertain atmospheric environments.
Through its innovative framework, SFM successfully addresses the persistent issue of reconciling low and high-resolution data in atmospheric modeling, achieving a careful balance between deterministic and stochastic elements. By providing high-fidelity downscaling, SFM opens up new possibilities for advanced meteorological simulations, supporting improved climate resilience and localized weather predictions. The SFM method marks a meaningful advancement in atmospheric science, setting a new benchmark in model accuracy for high-resolution weather data, especially when conventional models face limitations due to data scarcity and resolution misalignment.
Check out the Paper. All credit for this research goes to the researchers of this project. Also, don’t forget to follow us on Twitter and join our Telegram Channel and LinkedIn Group. If you like our work, you will love our newsletter.. Don’t Forget to join our 55k+ ML SubReddit.
[Sponsorship Opportunity with us] Promote Your Research/Product/Webinar with 1Million+ Monthly Readers and 500k+ Community Members
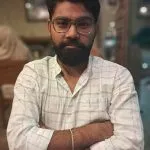
Nikhil is an intern consultant at Marktechpost. He is pursuing an integrated dual degree in Materials at the Indian Institute of Technology, Kharagpur. Nikhil is an AI/ML enthusiast who is always researching applications in fields like biomaterials and biomedical science. With a strong background in Material Science, he is exploring new advancements and creating opportunities to contribute.