Image by Author
Â
MCP, or Model Context Protocol, is a groundbreaking framework that is rapidly gaining traction in the AI and large language model (LLM) community. It acts as a universal connector for AI systems, enabling seamless integration with external resources, APIs, and services. Think of MCP as a standardized protocol that allows LLMs to interact with tools and data sources in a consistent and efficient way, much like how USB-C works for devices.
In this tutorial, we will build our own MCP server using the Yahoo Finance Python API to fetch real-time stock prices, compare them, and provide historical analysis. This project is beginner-friendly, meaning you only need a basic understanding of Python to complete this project.
Â
1. Setting up Environment for Using MCP Server
Â
First, go to the claude.ai website and download and install Claude Desktops. Then, install the MCP and YFinanace Python package using the PIP command.
$ pip install "mcp[cli]" yfinance
Â
2. Building MCP Server
Â
Create the project directory with a project name of your choice, and then create the stock_price_server.py
Python file and add the following code.
- MCP Server Initialization: Creates a customizable MCP server named “Stock Price Server” using the FastMCP class.
- Stock Price Retrieval: The
get_stock_price
function fetches the latest stock price for a specified ticker symbol, with fallback options for market closures. - Resource Exposure: The
stock_resource
function formats and exposes stock price data as a resource, providing user-friendly output. - Historical Data Retrieval: The
get_stock_history
function retrieves and returns historical stock data as a CSV formatted string for a specified period. - Stock Comparison: The
compare_stocks
function compares the prices of two ticker symbols and returns a formatted message indicating their relative values. - Error Handling: Each function includes robust error handling to return meaningful messages when data retrieval fails.
from mcp.server.fastmcp import FastMCP
import yfinance as yf
# Create an MCP server with a custom name
mcp = FastMCP("Stock Price Server")
@mcp.tool()
def get_stock_price(symbol: str) -> float:
"""
Retrieve the current stock price for the given ticker symbol.
Returns the latest closing price as a float.
"""
try:
ticker = yf.Ticker(symbol)
# Get today's historical data; may return empty if market is closed or symbol is invalid.
data = ticker.history(period="1d")
if not data.empty:
# Use the last closing price from today's data
price = data['Close'].iloc[-1]
return float(price)
else:
# As a fallback, try using the regular market price from the ticker info
info = ticker.info
price = info.get("regularMarketPrice", None)
if price is not None:
return float(price)
else:
return -1.0 # Indicate failure
except Exception:
# Return -1.0 to indicate an error occurred when fetching the stock price
return -1.0
@mcp.resource("stock://{symbol}")
def stock_resource(symbol: str) -> str:
"""
Expose stock price data as a resource.
Returns a formatted string with the current stock price for the given symbol.
"""
price = get_stock_price(symbol)
if price str:
"""
Retrieve historical data for a stock given a ticker symbol and a period.
Returns the historical data as a CSV formatted string.
Parameters:
symbol: The stock ticker symbol.
period: The period over which to retrieve historical data (e.g., '1mo', '3mo', '1y').
"""
try:
ticker = yf.Ticker(symbol)
data = ticker.history(period=period)
if data.empty:
return f"No historical data found for symbol '{symbol}' with period '{period}'."
# Convert the DataFrame to a CSV formatted string
csv_data = data.to_csv()
return csv_data
except Exception as e:
return f"Error fetching historical data: {str(e)}"
@mcp.tool()
def compare_stocks(symbol1: str, symbol2: str) -> str:
"""
Compare the current stock prices of two ticker symbols.
Returns a formatted message comparing the two stock prices.
Parameters:
symbol1: The first stock ticker symbol.
symbol2: The second stock ticker symbol.
"""
price1 = get_stock_price(symbol1)
price2 = get_stock_price(symbol2)
if price1 price2:
result = f"{symbol1} (${price1:.2f}) is higher than {symbol2} (${price2:.2f})."
elif price1
Â
3. Inspecting the MCP Server
Â
We will now test our server using the MCP Server Inspector by running the following command:
$ mcp dev stock_price_server.py
Â
This command will redirect you to the URL where the MCP Inspector is serving. Using the user interface, you can test various tools and ensure everything is functioning as intended.
This server now exposes:
get_stock_price
: A tool to fetch the latest pricestock_resource
: A resource that returns a human-readable price messageget_stock_history
: A tool that returns historical data as CSVcompare_stocks
: A tool to compare the current prices of two stocks
Â
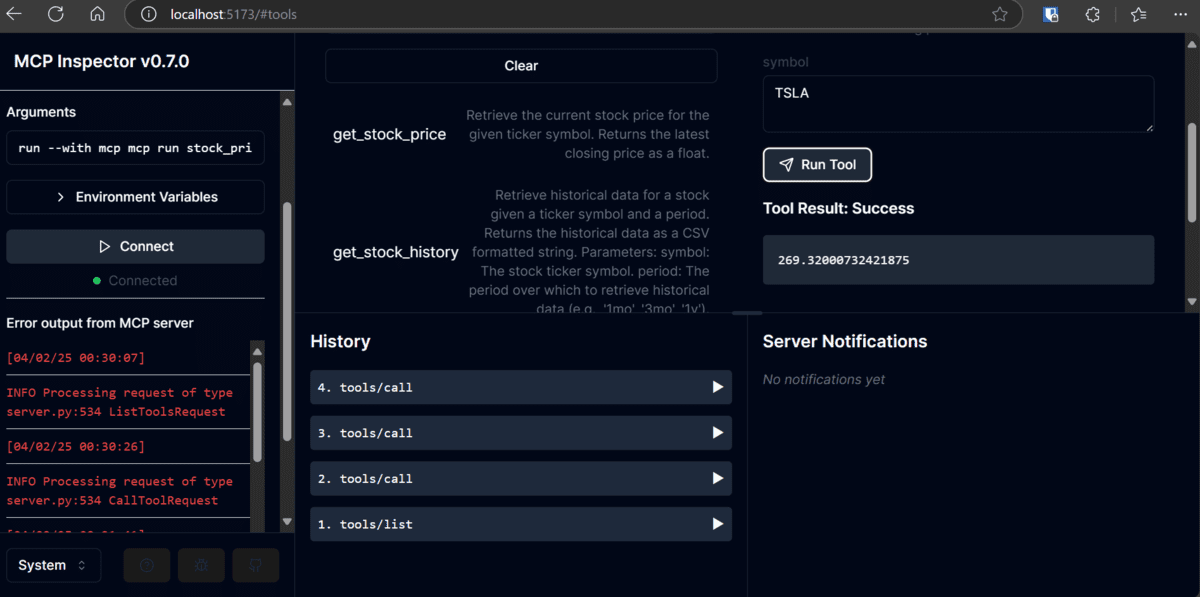
Â
4. Setting Up MCP server for Claude Desktop
Â
Once we are satisfied with our MCP server, we will integrate it into Claude Desktop by running the following command in the terminal:Â Â
$ mcp install stock_price_server.py --name "Stock Price Server"
Â
When you relaunch Claude Desktop, you will see new icons below the chat option. This indicates that our MCP is configured. You can click on these icons to check how many tools are available for Claude Desktop.
Â
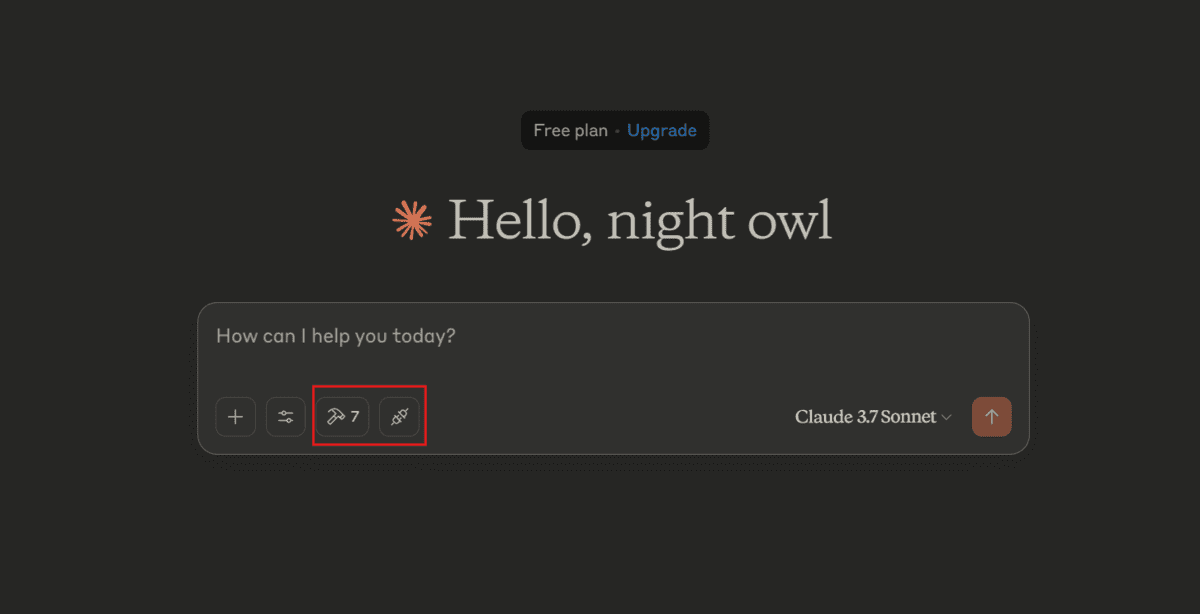
Â
5. Testing the MCP server with Claude Desktop
Â
We will begin by asking Claude Desktop to compare two stock prices.Â
Â
Prompt: “Could you please compare the prices of Microsoft and Tesla stocks?”
Â
After you enter the prompt, it will request permission to use the specified tool. Just click on the button that says “Allow for This Chat.”
Â

Â
We have a simple comparison of the prices of Microsoft and Tesla.
Â
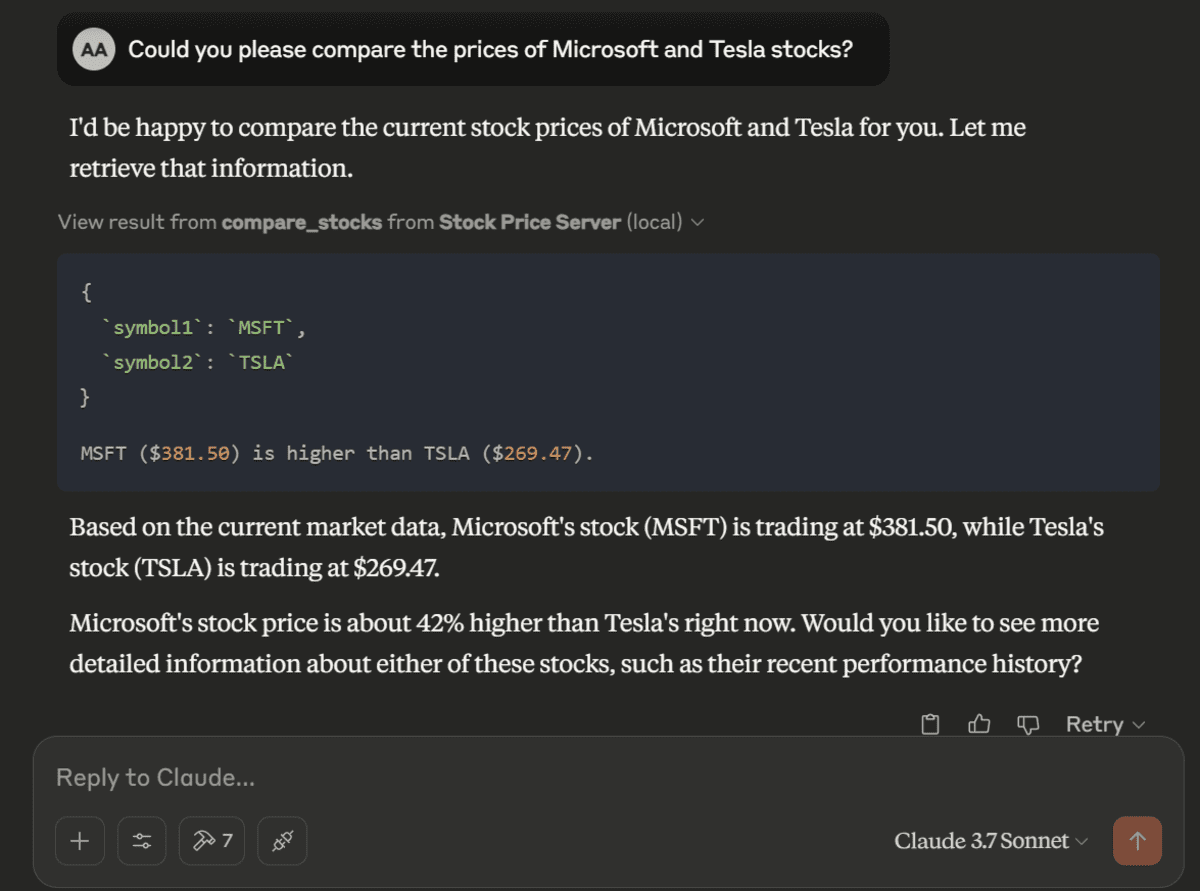
Â
We will now request a historical analysis of Tesla stocks for the past month.Â
Â
Prompt: “Could you please provide the historical data for Tesla over the past month?”
Â
Once again, we received an excellent analysis, even better than if I had manually upload the CSV file.
Â

Â
Final Thoughts
Â
MCP is a new concept that builds on traditional ideas such as function calling and AI agents. In the future, we aim to perfect these technologies to achieve seamless integration of external resources with your AI. As a result, upon your request, it will be able to perform various tasks such as ordering groceries, sending emails, creating Spotify playlists, and sharing them with your friends.
Â
Â
Abid Ali Awan (@1abidaliawan) is a certified data scientist professional who loves building machine learning models. Currently, he is focusing on content creation and writing technical blogs on machine learning and data science technologies. Abid holds a Master’s degree in technology management and a bachelor’s degree in telecommunication engineering. His vision is to build an AI product using a graph neural network for students struggling with mental illness.