Mental illness is a critical global public health issue, with one in eight people affected and many lacking access to adequate treatment. A significant challenge in mental health professional training is the disconnect between formal education and real-world patient interactions. A study interviewed 12 experts to address this, revealing that traditional role-playing is often unrealistic and insufficient for preparing trainees. Leveraging advancements in LLMs like ChatGPT, researchers propose using LLMs to create simulated patients. This approach aims to improve training fidelity and effectiveness, helping bridge the gap between current training methods and the complexities of real-life therapy.
Researchers from Carnegie Mellon, Princeton, Pittsburgh, and Stanford have developed PATIENT-Ψ, an innovative patient simulation framework for CBT training. PATIENT-Ψ uses cognitive models based on CBT principles and integrates them with LLMs to simulate therapy patients. The interactive training framework, PATIENT-Ψ-TRAINER, allows trainees to practice forming cognitive models through simulated therapy sessions. A study involving 33 experts and trainees showed that PATIENT-Ψ closely mimics real patients and enhances trainees’ skills and confidence beyond traditional methods. PATIENT-Ψ-TRAINER offers customized training with diverse patient models and conversational styles, providing significant advantages over existing training approaches.
The development of PATIENT-Ψ is closely linked to the recent application of LLMs in fields such as psychology, education, and computational social science. Unlike existing research on LLMs in cognitive behavioral therapy (CBT), which primarily addresses cognitive distortion detection and negative thought reframing, PATIENT-Ψ focuses on creating realistic and interactive simulations for CBT professional training. This work aligns with studies using LLMs for training in communication skills, emotion management, and clinical diagnosis. PATIENT-Ψ uniquely grounds its simulations in CBT principles, incorporates feedback mechanisms, and evaluates effectiveness with mental health professionals and trainees.
Constructing PATIENT-Ψ involves integrating cognitive models from CBT with LLMs to simulate realistic patient interactions. PATIENT-Ψ-CM, a dataset of 106 cognitive models created by clinical psychologists, serves as the foundation. These models cover diverse scenarios and conversational styles, reflecting various emotional and cognitive states. PATIENT-Ψ-TRAINER is an interactive framework for mental health trainees to practice formulating cognitive models through simulated therapy sessions. Trainees engage with PATIENT-Ψ, develop cognitive models, and receive feedback by comparing their formulations with the original models, facilitating self-guided, effective skill development.
PATIENT-Ψ’s realism was assessed by experts who compared it to traditional methods and a GPT-4 baseline. PATIENT-Ψ significantly outperformed both, closely mimicking real patients across emotional states, conversational styles, and maladaptive thoughts. Experts highlighted its ability to provide a realistic challenge in information extraction, unlike the overly responsive GPT-4 baseline. Additionally, PATIENT-Ψ accurately reflects its cognitive model components, offering trainees high-quality feedback. PATIENT-Ψ-TRAINER was deemed more effective for skill development and boosting confidence, making it a superior training tool for preparing mental health professionals.
PATIENT-Ψ is an innovative simulated patient merging cognitive models with an LLM to replicate real patient interactions. PATIENT-Ψ-TRAINER facilitates role-playing therapy sessions, where trainees formulate underlying cognitive models. User studies with mental health experts and trainees confirm PATIENT-Ψ’s fidelity and the effectiveness of PATIENT-Ψ-TRAINER, surpassing traditional methods and a GPT-4 baseline. The framework shows promise for transforming mental health professional training and can be adapted to broader therapy paradigms. However, the study acknowledges limitations in its current evaluation, suggesting future research could include longitudinal RCTs to measure skill improvements objectively.
Check out the Paper. All credit for this research goes to the researchers of this project. Also, don’t forget to follow us on Twitter.
Join our Telegram Channel and LinkedIn Group.
If you like our work, you will love our newsletter..
Don’t Forget to join our 45k+ ML SubReddit
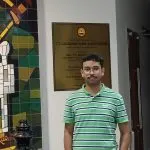
Sana Hassan, a consulting intern at Marktechpost and dual-degree student at IIT Madras, is passionate about applying technology and AI to address real-world challenges. With a keen interest in solving practical problems, he brings a fresh perspective to the intersection of AI and real-life solutions.