Developing an accurate differential diagnosis (DDx) is a fundamental part of medical care, typically achieved through a step-by-step process that integrates patient history, physical exams, and diagnostic tests. With the rise of LLMs, there’s growing potential to support and automate parts of this diagnostic journey using interactive, AI-powered tools. Unlike traditional AI systems focusing on producing a single diagnosis, real-world clinical reasoning involves continuously updating and evaluating multiple diagnostic possibilities as more patient data becomes available. Although deep learning has successfully generated DDx across fields like radiology, ophthalmology, and dermatology, these models generally lack the interactive, conversational capabilities needed to engage effectively with clinicians.
The advent of LLMs offers a new avenue for building tools that can support DDx through natural language interaction. These models, including general-purpose ones like GPT-4 and medical-specific ones like Med-PaLM 2, have shown high performance on multiple-choice and standardized medical exams. While these benchmarks initially assess a model’s medical knowledge, they don’t reflect its usefulness in real clinical settings or its ability to assist physicians during complex cases. Although some recent studies have tested LLMs on challenging case reports, there’s still a limited understanding of how these models might enhance clinician decision-making or improve patient care through real-time collaboration.
Researchers at Google introduced AMIE, a large language model tailored for clinical diagnostic reasoning, to evaluate its effectiveness in assisting with DDx. AMIE’s standalone performance outperformed unaided clinicians in a study involving 20 clinicians and 302 complex real-world medical cases. When integrated into an interactive interface, clinicians using AMIE alongside traditional tools produced significantly more accurate and comprehensive DDx lists than those using standard resources alone. AMIE not only improved diagnostic accuracy but also enhanced clinicians’ reasoning abilities. Its performance also surpassed GPT-4 in automated evaluations, showing promise for real-world clinical applications and broader access to expert-level support.
AMIE, a language model fine-tuned for medical tasks, demonstrated strong performance in generating DDx. Its lists were rated highly for quality, appropriateness, and comprehensiveness. In 54% of cases, AMIE’s DDx included the correct diagnosis, outperforming unassisted clinicians significantly. It achieved a top-10 accuracy of 59%, with the proper diagnosis ranked first in 29% of cases. Clinicians assisted by AMIE also improved their diagnostic accuracy compared to using search tools or working alone. Despite being new to the AMIE interface, clinicians used it similarly to traditional search methods, showing its practical usability.
In a comparative analysis between AMIE and GPT-4 using a subset of 70 NEJM CPC cases, direct human evaluation comparisons were limited due to different sets of raters. Instead, an automated metric that was shown to align reasonably with human judgment was used. While GPT-4 marginally outperformed AMIE in top-1 accuracy (though not statistically significant), AMIE demonstrated superior top-n accuracy for n > 1, with notable gains for n > 2. This suggests that AMIE generated more comprehensive and appropriate DDx, a crucial aspect in real-world clinical reasoning. Additionally, AMIE outperformed board-certified physicians in standalone DDx tasks and significantly improved clinician performance as an assistive tool, yielding higher top-n accuracy, DDx quality, and comprehensiveness than traditional search-based assistance.
Beyond raw performance, AMIE’s conversational interface was intuitive and efficient, with clinicians reporting increased confidence in their DDx lists after its use. While limitations exist—such as AMIE’s lack of access to images and tabular data in clinician materials and the artificial nature of CPC-style case presentations the model’s potential for educational support and diagnostic assistance is promising, particularly in complex or resource-limited settings. Nonetheless, the study emphasizes the need for careful integration of LLMs into clinical workflows, with attention to trust calibration, the model’s uncertainty expression, and the potential for anchoring biases and hallucinations. Future work should rigorously evaluate AI-assisted diagnosis’s real-world applicability, fairness, and long-term impacts.
Check out Paper. All credit for this research goes to the researchers of this project. Also, feel free to follow us on Twitter and don’t forget to join our 85k+ ML SubReddit.
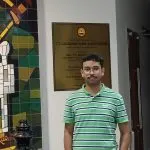
Sana Hassan, a consulting intern at Marktechpost and dual-degree student at IIT Madras, is passionate about applying technology and AI to address real-world challenges. With a keen interest in solving practical problems, he brings a fresh perspective to the intersection of AI and real-life solutions.
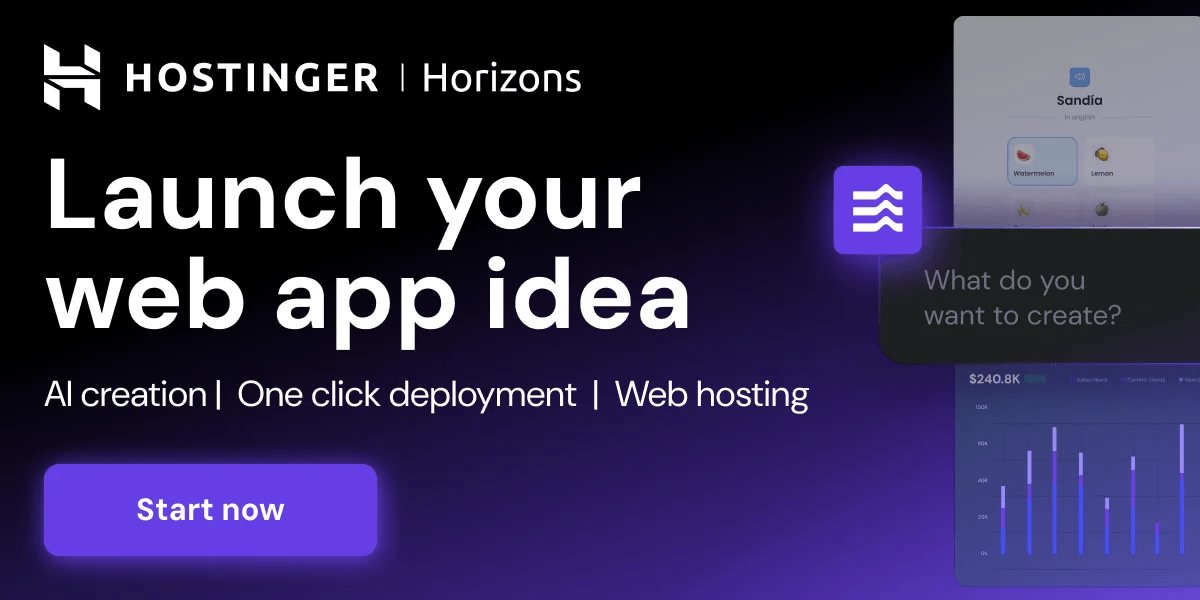