Medical abstractive summarization faces challenges in balancing faithfulness and informativeness, often compromising one for the other. While recent techniques like in-context learning (ICL) and fine-tuning have enhanced summarization, they frequently overlook key aspects such as model reasoning and self-improvement. The lack of a unified benchmark complicates systematic evaluation due to inconsistent metrics and datasets. The stochastic nature of LLMs can lead to summaries that deviate from input documents, posing risks in medical contexts where accurate and complete information is vital for decision-making and patient outcomes.
Researchers from ASUS Intelligent Cloud Services, Imperial College London, Nanyang Technological University, and Tan Tock Seng Hospital have developed a comprehensive benchmark for six advanced abstractive summarization methods across three datasets using five standardized metrics. They introduce uMedSum, a modular hybrid framework designed to enhance faithfulness and informativeness by sequentially removing confabulations and adding missing information. uMedSum significantly outperforms previous GPT-4-based methods, achieving an 11.8% improvement in reference-free metrics and preferred by doctors 6 times more in complex cases. Their contributions include an open-source toolkit to advance medical summarization research.
Summarization typically involves extractive methods that select key phrases from the input text and abstractive methods that rephrase content for clarity. Recent advances include semantic matching, keyphrase extraction using BERT, and reinforcement learning for factual consistency. However, most approaches use either extractive or abstractive methods in isolation, limiting effectiveness. Confabulation detection remains challenging, as existing techniques often fail to remove ungrounded information accurately. To address these issues, a new framework integrates extractive and abstractive methods to remove confabulations and add missing information, achieving a better balance between faithfulness and informativeness.
To address the lack of a benchmark in medical summarization, the uMedSum framework evaluates four recent methods, including Element-Aware Summarization and Chain of Density, integrating the best-performing techniques for initial summary generation. The framework then removes confabulations using Natural Language Inference (NLI) models, which detect and eliminate inaccurate information by breaking summaries into atomic facts. Finally, missing key information is added to enhance the summary’s completeness. This three-stage, modular process ensures that summaries are both faithful and informative, improving existing state-of-the-art medical summarization methods.
The study assesses state-of-the-art medical summarization methods, enhancing top-performing models with the uMedSum framework. It uses three datasets: MIMIC III (Radiology Report Summarization), MeQSum (Patient Question Summarization), and ACI-Bench (doctor-patient dialogue summarization), evaluated with both reference-based and reference-free metrics. Among the four benchmarked models—LLaMA3 (8B), Gemma (7B), Meditron (7B), and GPT-4—GPT-4 consistently outperformed others, particularly with ICL. The uMedSum framework notably improved performance, especially in maintaining factual consistency and informativeness, with seven of the top ten methods incorporating uMedSum.
In conclusion, uMedSum is a framework that significantly improves medical summarization by addressing the challenges of maintaining faithfulness and informativeness. Through a comprehensive benchmark of six advanced summarization methods across three datasets, uMedSum introduces a modular approach for removing confabulations and adding missing key information. This approach leads to an 11.8% improvement in reference-free metrics compared to previous state-of-the-art (SOTA) methods. Human evaluations reveal doctors prefer uMedSum’s summaries six times more than previous methods, especially in challenging cases. uMedSum sets a new standard for accurate and informative medical summarization.
Check out the Paper. All credit for this research goes to the researchers of this project. Also, don’t forget to follow us on Twitter and join our Telegram Channel and LinkedIn Group. If you like our work, you will love our newsletter..
Don’t Forget to join our 50k+ ML SubReddit
Find Upcoming AI Webinars here
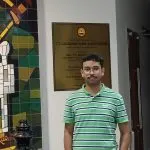
Sana Hassan, a consulting intern at Marktechpost and dual-degree student at IIT Madras, is passionate about applying technology and AI to address real-world challenges. With a keen interest in solving practical problems, he brings a fresh perspective to the intersection of AI and real-life solutions.