The deep learning model of Stable Diffusion is huge. The weight file is multiple GB large. Retraining the model means to update a lot of weights and that is a lot of work. Sometimes we must modify the Stable Diffusion model, for example, to define a new interpretation of prompts or make the model to generate a different style of painting by default. Indeed there are ways to make such an extension to existing model without modifying the existing model weights. In this post, you will learn about the low-rank adaptation, which is the most common technique for modifying the behavior of Stable Diffusion.
Let’s get started.
Using LoRA in Stable Diffusion
Photo by Agent J. Some rights reserved.
Overview
This post is in three parts; they are:
- What Is Low-Rank Adaptation
- Checkpoint or LoRA?
- Examples of LoRA Models
What Is Low-Rank Adaptation
LoRA, or Low-Rank Adaptation, is a lightweight training technique used for fine-tuning Large Language and Stable Diffusion Models without needing full model training. Full fine-tuning of larger models (consisting of billions of parameters) is inherently expensive and time-consuming. LoRA works by adding a smaller number of new weights to the model for training, rather than retraining the entire parameter space of the model. This significantly reduces the number of trainable parameters, allowing for faster training times and more manageable file sizes (typically around a few hundred megabytes). This makes LoRA models easier to store, share, and use on consumer GPUs.
In simpler terms, LoRA is like adding a small team of specialized workers to an existing factory, rather than building an entirely new factory from scratch. This allows for more efficient and targeted adjustments to the model.
LoRA is a state-of-the-art fine-tuning method proposed by Microsoft researchers to adapt larger models to particular concepts. A typical complete fine-tuning involves updating the weights of the entire model in each dense layer of the neural network. Aghajanyan et al.(2020) explained that pre-trained over-parametrized models actually reside on a low intrinsic dimension. LoRA approach is based on this finding, by by restricting weight updates to the residual of the model.
Suppose that $W_0\in \mathbb{R}^{d\times k}$ represents a pretrained weight matrix of size $\mathbb{R}^{d\times k}$ (i.e., a matrix of $d$ rows and $k$ columns in real numbers), and it changes by $\Delta W$ (the update matrix) such that the fine-tuned model’s weight are
$$ W’ = W_0 + \Delta W$$
LoRA use the technique lowers the rank of this update matrix $\Delta W$ by rank decomposition such that:
$$
\Delta W = B \times A
$$
where $B\in\mathbb{R}^{d\times r}$ and $A\in\mathbb{R}^{r\times k}$, such that $r\ll \min(k,d)$$.
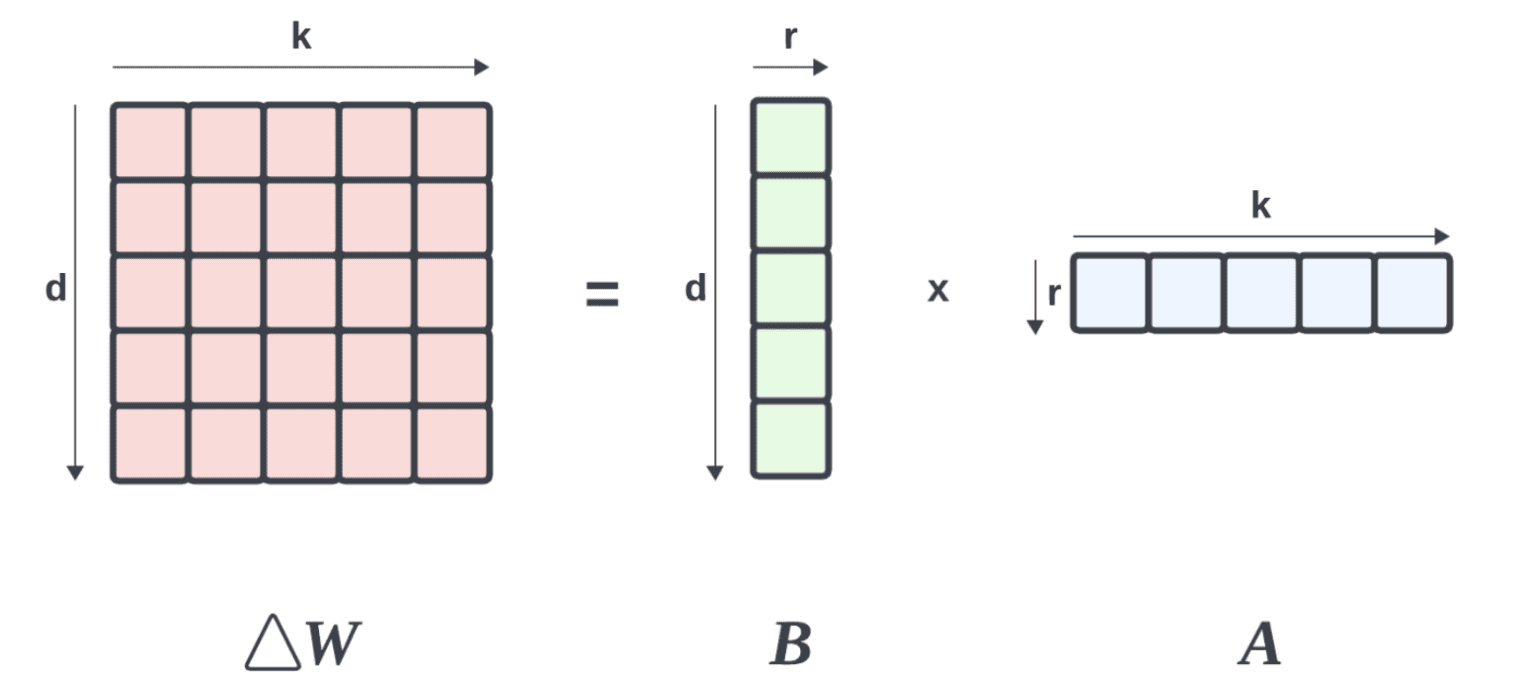
Breaking a matrix into two lower rank matrices
By freezing $W_0$ (to save memory), we can fine-tune $A$ and $B$, which contain the trainable parameters for adaptation. This results in the fine-tuned model’s forward pass looking like this:
$$
h = W’x = W_0 x + BA x
$$
For Stable diffusion fine-tuning, it’s sufficient to apply rank decomposition to cross-attention layers (shaded below) which are responsible for integrating the prompt and image information. Specifically, the weight matrices $W_O$, $W_Q$, $W_K$, and $W_V$ in these layers are decomposed to lower the rank of the weight updates. By freezing other MLP modules and fine-tuning only the decomposed matrices $A$ and $B$, LoRA models can lead to smaller file sizes while being much faster.
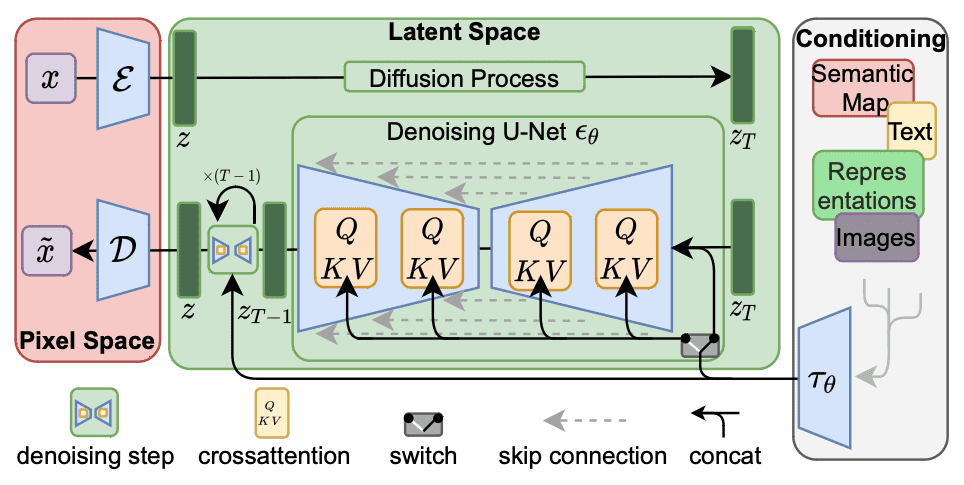
Workflow of Stable Diffusion. The crossattention modules can be modified by LoRA.
Checkpoint or LoRA?
A checkpoint model is a complete, pre-trained model saved at a particular state during training. It contains all the parameters learned during training and can be used for inference or fine-tuning. However, fine-tuning a checkpoint model requires updating all the weights in the model, which can be computationally expensive and result in large file sizes (typically in several GBs for Stable Diffusion).
On the other hand, LoRA (Low-Rank Adaptation) models are much smaller and more efficient. It behaves as an adapter that builds on the top of a checkpoint model (foundation or base model). LoRA models update only a subset of a checkpoint model’s parameters (enhancing a checkpoint model). This enables these models to be small-sized (usually 2MB to 500MB) and be frequently fine-tuned for specific concepts or styles.
For example, fine-tuning a Stable Diffusion model may be done with DreamBooth. DreamBooth is a fine-tuning method that updates the entire model to adapt to a specific concept or style. While it can produce impressive results, it comes with a significant drawback: the size of the fine-tuned model. Since DreamBooth updates the entire model, the resulting checkpoint model can be quite large (approximately 2 to 7 GBs) and require a lot of GPU resources for training. In contrast, A LoRA model significantly requires less GPU requirements yet the inferences are still comparable to those of a Dreamboothed checkpoint.
While it is the most common, LoRA is not the only way to modify Stable Diffusion. Refer to the workflow as illustrated above, the crossattention module took input $\tau_\theta$, which usually resulted from converting the prompt text into text embeddings. Modifying the embedding is what Text Inversions do to change the behavior of Stable Diffusion. Textual Inversions is even smaller and faster than LoRA. However, Textual Inversions have a limitation: they only fine-tune the text embeddings for a particular concept or style. The U-Net, which is responsible for generating the images, remains unchanged. This means that Textual Inversions can only generate images that are similar to the ones it was trained on and cannot produce anything beyond what it already knows.
Examples of LoRA models
There are many different LoRA models within the context of Stable Diffusion. One way to categorize them is to base on what the LoRA model does:
- Character LoRA: These models are fine-tuned to capture the appearance, body proportions, and expressions of specific characters, often found in cartoons, video games, or other forms of media. They are useful for creating fan artwork, game development, and animation/illustration purposes.
- Style LoRA: These models are fine-tuned on artwork from specific artists or styles to generate images in that style. They are often used to stylize a reference image in a particular aesthetic.
- Clothing LoRA: These models are fine-tuned on artwork from specific artists or styles to generate images in that style. They are often used to stylize a reference image in a particular aesthetic.
Some examples are as follows:
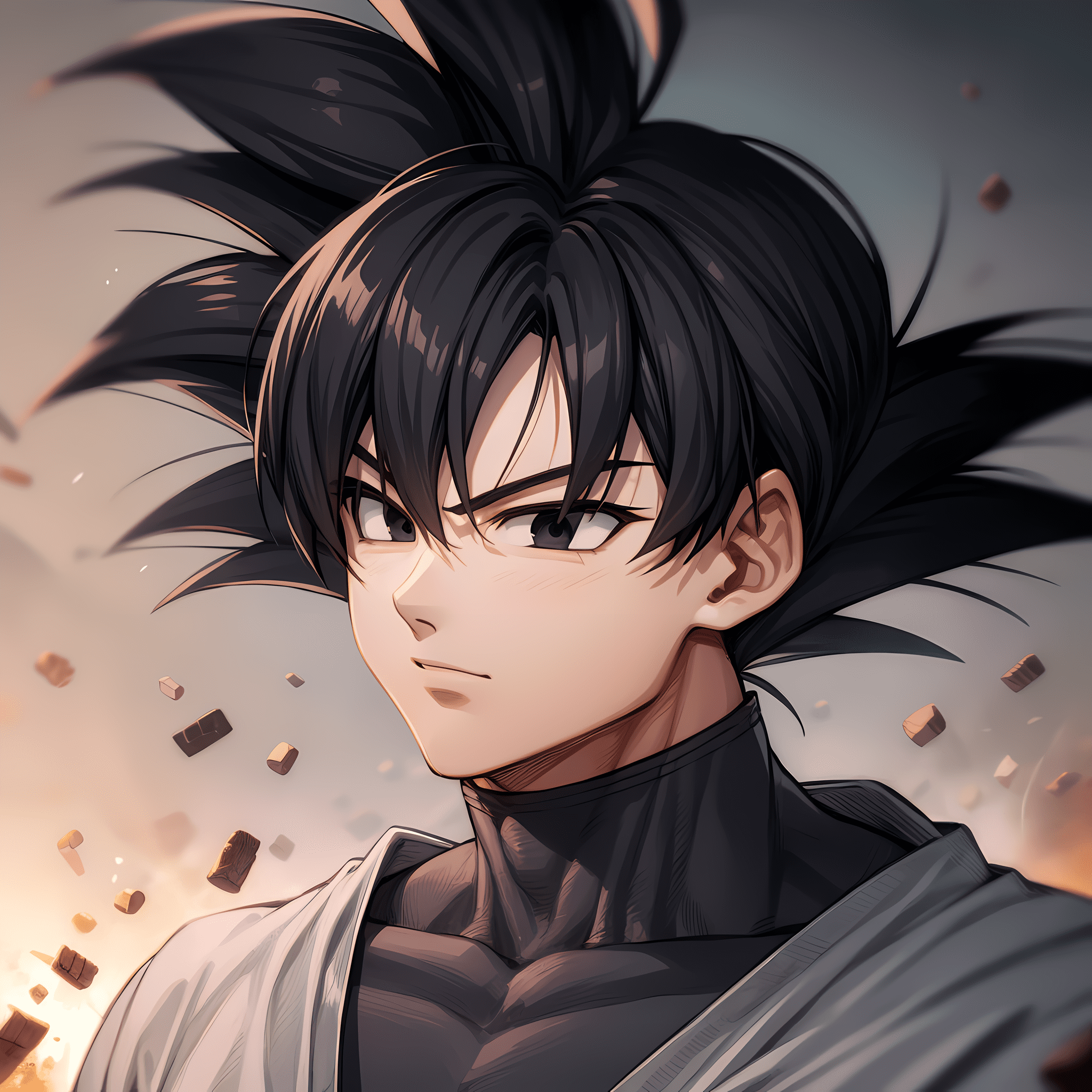
Image created with character LoRA “goku black [dragon ball super]” on Civitai, authored by TheGooder
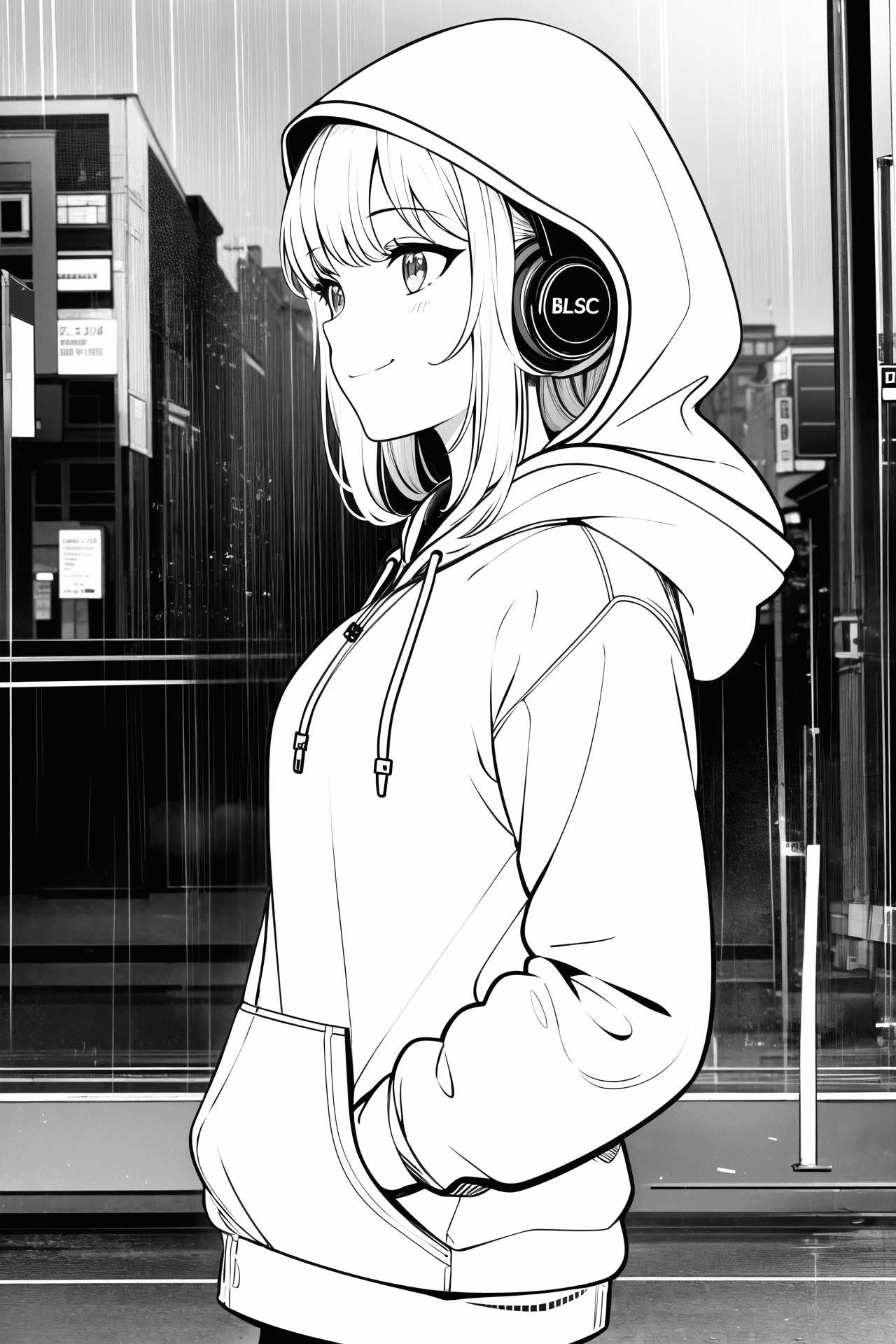
Image created with style LoRA “Anime Lineart / Manga-like (线稿/線画/マンガ風/漫画风) Style” on Civitai, authored by CyberAIchemist.
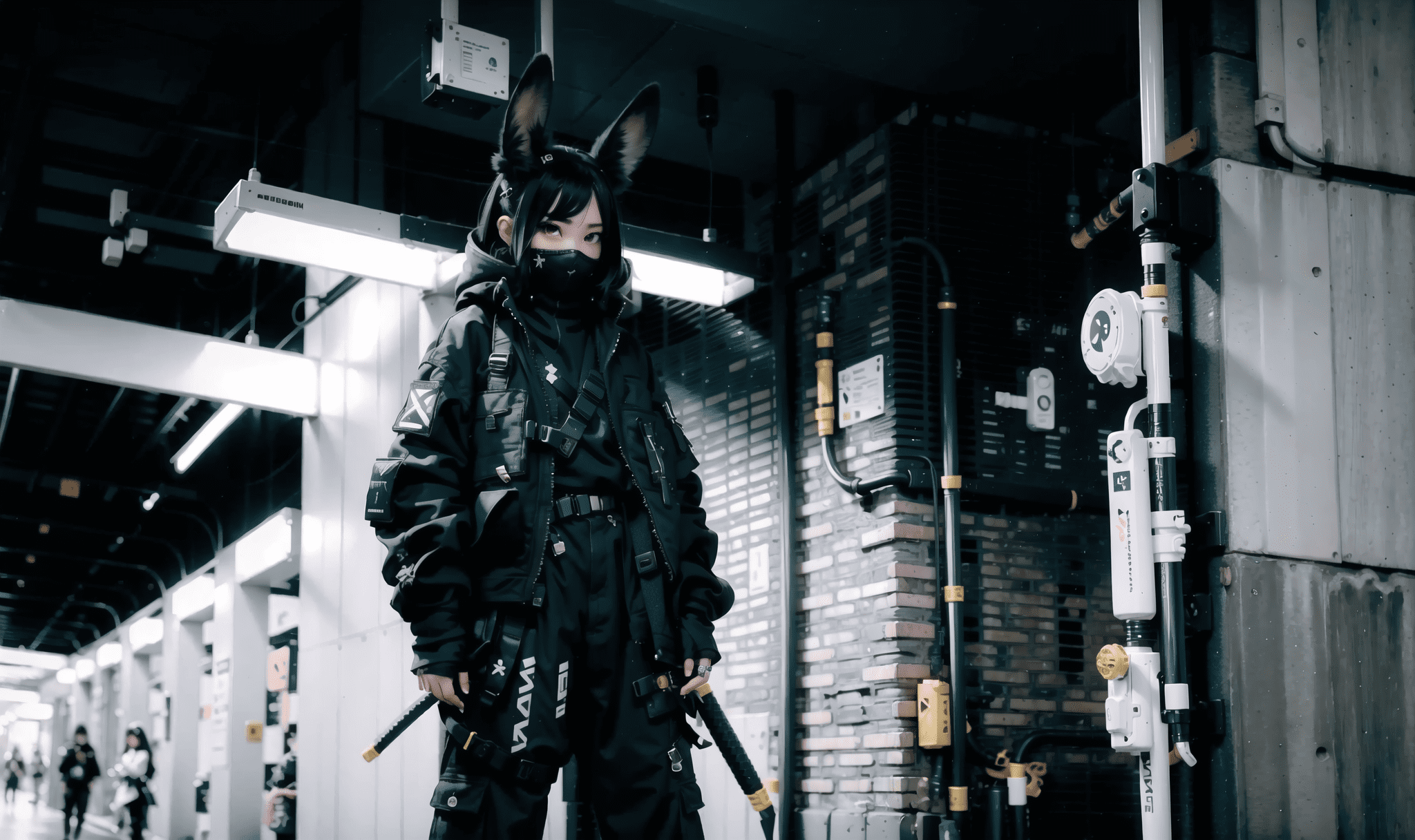
Image created with clothing LoRA “Anime Lineart / Manga-like (线稿/線画/マンガ風/漫画风) Style” on Civitai, authored by YeHeAI.
The most popular place to find LoRA model files is on Civitai. If you are using the Stable Diffusion Web UI, all you need to do is to download the model file and put it into the folder stable-diffusion-webui/models/Lora
.
To use the LoRA from the Web UI, you just need to add the name of the LoRA in angle brackets as part of your prompt. For example, one of the image above is generated with the prompt:
best masterpiece,1girl,solo,incredibly absurdres,hoodie,headphones, street,outdoors,rain,neon lights, light smile, hood up, hands in pockets, looking away, from side, lineart, monochrome, <lora:animeoutlineV4_16:1>
The part “<lora:animeoutlineV4_16:1>” means to use the LoRA which the model file is named as animeoutlineV4_16.safetensors
, and apply it with weight 1. Note that in the prompt, nothing mentioned about the line art style except the reference to a LoRA model. Hence you can see that the LoRA model produced an enormous effect to the output. If you are curious, you can often find the prompt and other parameters used to generate the picture from those posted on Civitai.
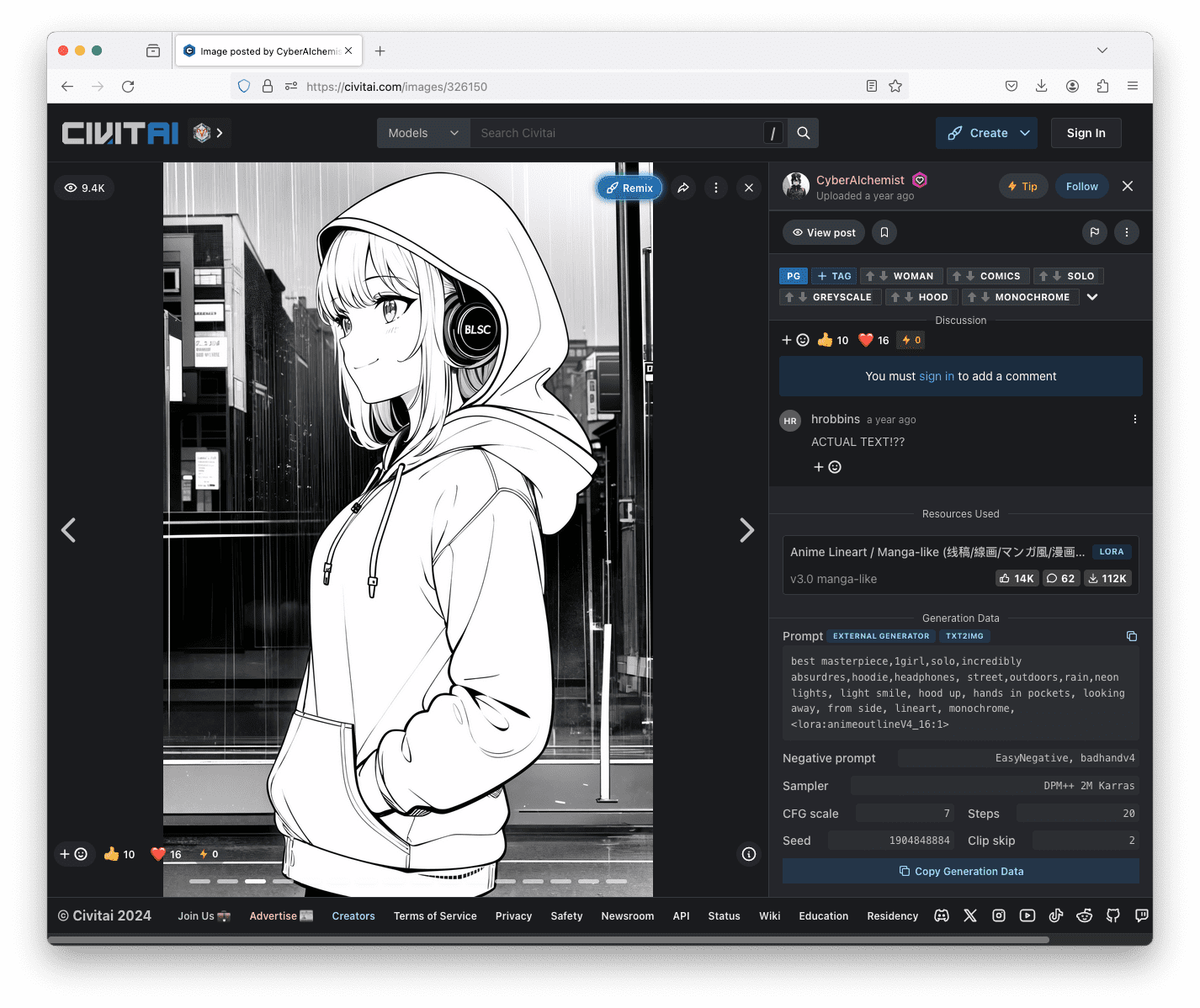
Checking out an image posted on Civitai can see the prompt and other parameters used to generate it on the right half of the screen.
As a final remark, LoRA depends on the model you used. For example, Stable Diffusion v1.5 and SD XL are incompatible in architecture so you need a LoRA that match the version of your base model.
Further Readings
Below are there papers introduced the LoRA fine-tuning techniques:
Summary
In this post, you learned what is LoRA in Stable Diffusion and why it is a lightweight enhancement. You also learned that using LoRA in Stable Diffusion Web UI is as easy as adding an extra keyword to the prompt There are many LoRA models developed by Stable Diffusion users and put up on the Internet for you to download. You can find one to easily change the generated result without much worrying on how to describe the style you want it to change.